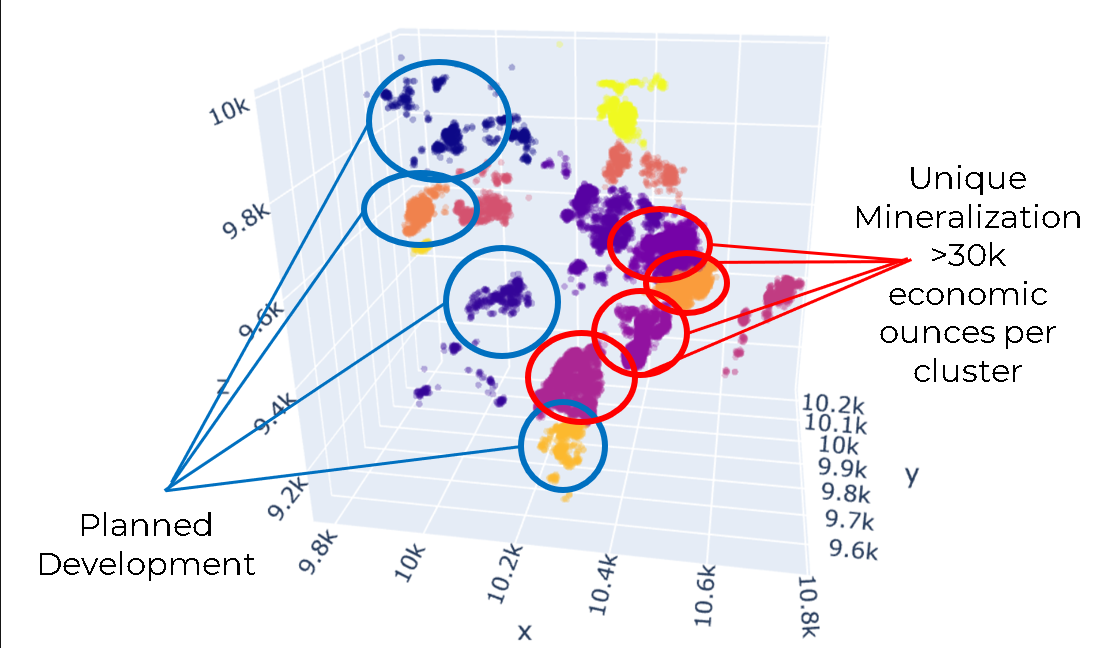
Fig. 1. Clusters of Au mineralization accessible to existing infrastructure where clusters in planned development are corroborated and unique clusters are verified using drill targets.
Predicting clusters of mineralization and optimally placed drilling targets for in-mine exploration is a valuable strategy to expand an asset’s reserve for reporting and planning purposes. In this paper, we propose a machine learning (ML)-based protocol that accepts drill hole data and chip data to produce extrapolated grade predictions for blocks outside the boundaries of resource model domains. We train a neural network using the assayed data from the mine site to predict clusters of gold mineralization outside of the data domain (i.e., make grade predictions for blocks with no assayed samples and outside the variography boundaries). We demonstrate the proposed method’s ability to find clusters of mineralization while reducing the noise from the nugget effect from live asset gold-grade data. While chip data bias and drill hole density introduces error to ML’s prediction, the results of the temporal benchmarking of data and live test with short-range drill targets to confirm the prediction clearly show the ML’s robustness against varying data densities and generalizability given the lack of overfitting (Fig. 1). It is notable that large clusters of mineralization were verified using optimally placed drill holes constrained by the mine’s underground infrastructure and existing stopes.